Introducing the #AISummer2023 series, a continuation of the original #30DayAIChallenge in Spring. As the field of AI evolves, this series delves deeper into the latest advancements in AI tools and capabilities. It represents not just my practical tinkering over the Summer, but a metaphorical ‘summer’ of AI growth and evolution.
Music has always been a fascinating subject for study, not just for its emotional impact, but also for the trends and patterns that drive its popularity. In this experiment with ChatGPT and Code Interpreter, we dive into a comprehensive archive that spans 1,095 Days (3 years) of Top 200 music charts from a popular Music Streaming Service, courtesy this dataset provided on Kaggle (while the original dataset runs from January 1, 2017 to May 29, 2023, I’ve only used the period from 30 May 2020 onwards for this test). Overall, the file contained 305,989 rows of data.
From examining the popularity of artists to analyzing the audio features that characterize these hit songs, we uncover some intriguing insights.
The Dataset
The dataset contains 21 richly detailed columns, offering everything from song rankings to artist nationality and even unique song IDs. Furthermore, the dataset has been enriched with audio features for each song, like:
- Danceability: Describes how suitable a track is for dancing
- Energy: Represents a perceptual measure of intensity and activity. Energetic tracks feel fast, loud and noisy.
- Loudness: The overall loudness of a track in decibels (dB)
- Speechiness: Detects the presence of spoken words in a track.
- Acousticness: Describes whether a track uses only or primarily instruments that produce sound through acoustic means.
- Instrumentalness: Predicts whether a track contains no vocals.
- Valence: Describes the musical positiveness of a track
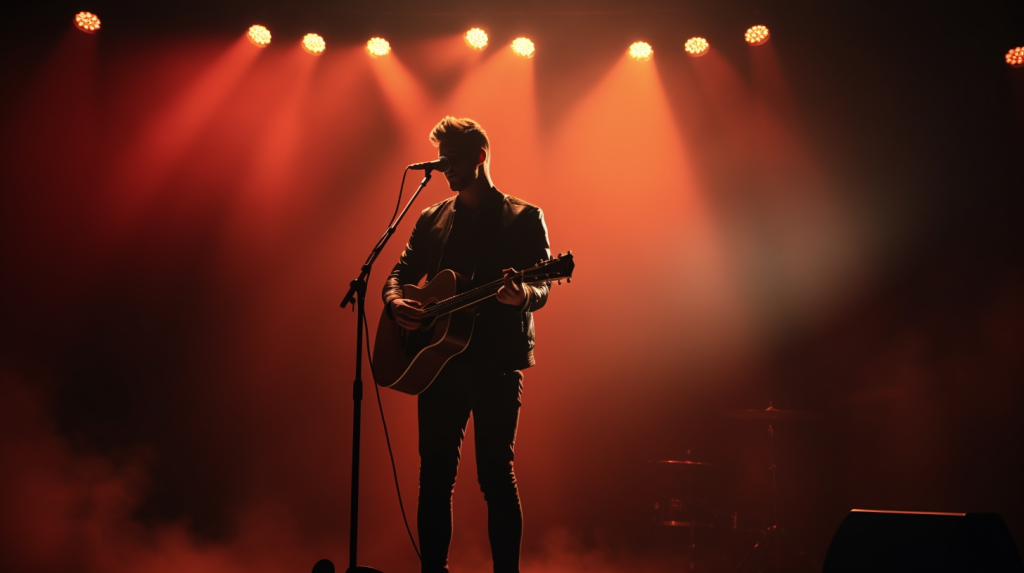
Who are the Top 10 Artists?
We could figure this out in a number of different ways…for example:
Top 10 Artists by Number of Appearances: This chart displays the artists who have appeared the most times in the Top 200 charts over the past three years. A higher number of appearances indicates consistent popularity and staying power.
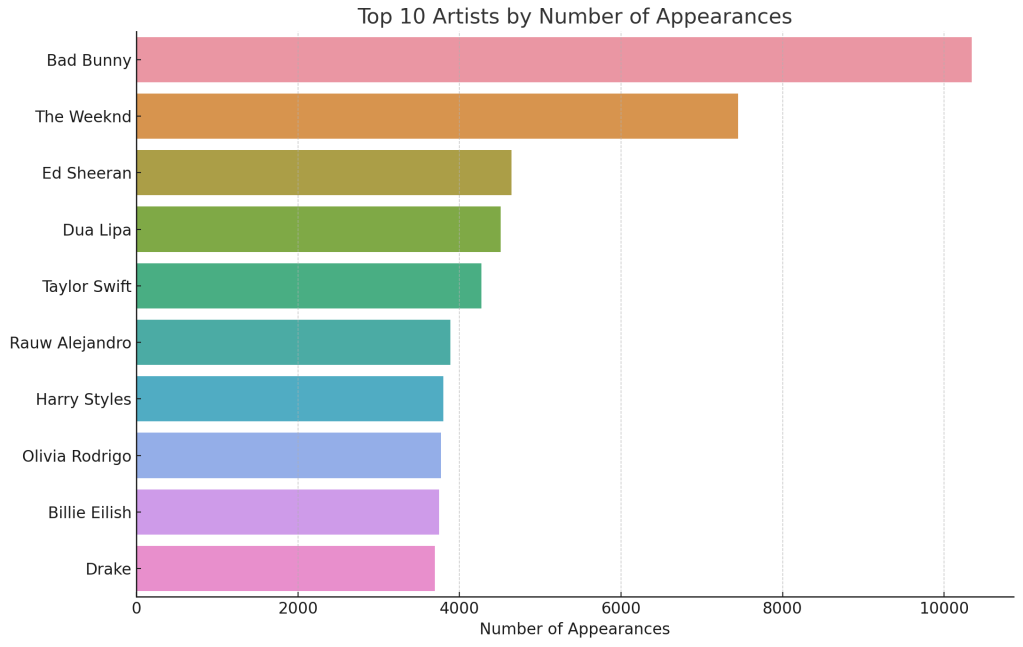
Here’s a bit more detail for the Top 20 overall:
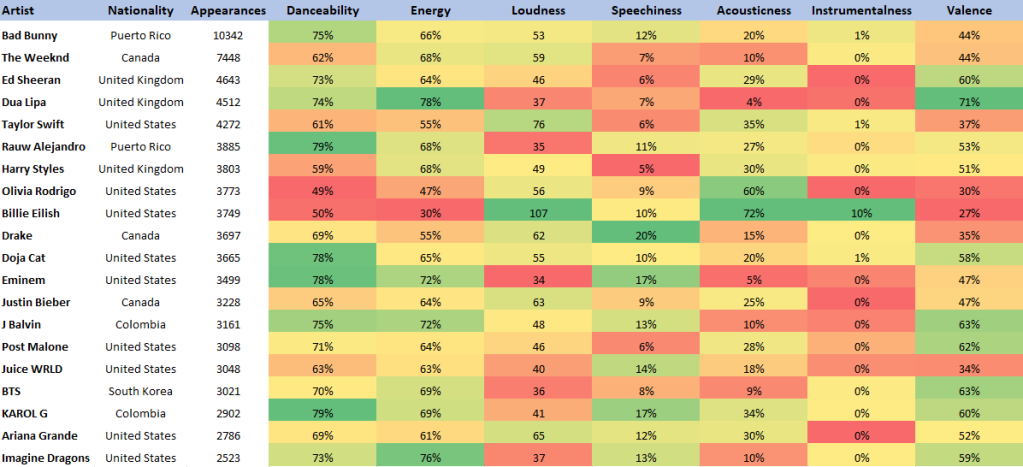
The Popularity Race: Artists on the Rise and the Fall
To figure out which artists have either grown in popularity or lost out over the past three years, we can employ several approaches:
1. Top 5 Artists by Improvement in Average Rank
This chart shows the top 5 artists who have shown the most improvement in their average rank over the past three years. A lower slope value indicates greater improvement.
2. Top 5 Artists by Increase in Appearances
This chart shows the top 5 artists who have increased their number of appearances in the Top 200 the most over the past three years. A higher slope indicates more frequent appearances.
3. Top 5 Artists by Increase in Total Points
This chart shows the top 5 artists who have increased their total points the most over the past three years. A higher slope indicates a greater increase in points.
4. Top 5 Improving Artists by Linear Regression
This chart uses linear regression on the rank data to identify the top 5 artists who have shown the most improvement over the past three years. A lower slope indicates improvement.
5. Top 5 Declining Artists by Linear Regression
Similarly, this chart identifies the top 5 artists who have declined the most in rank over the past three years, based on linear regression. A higher slope indicates a decline.
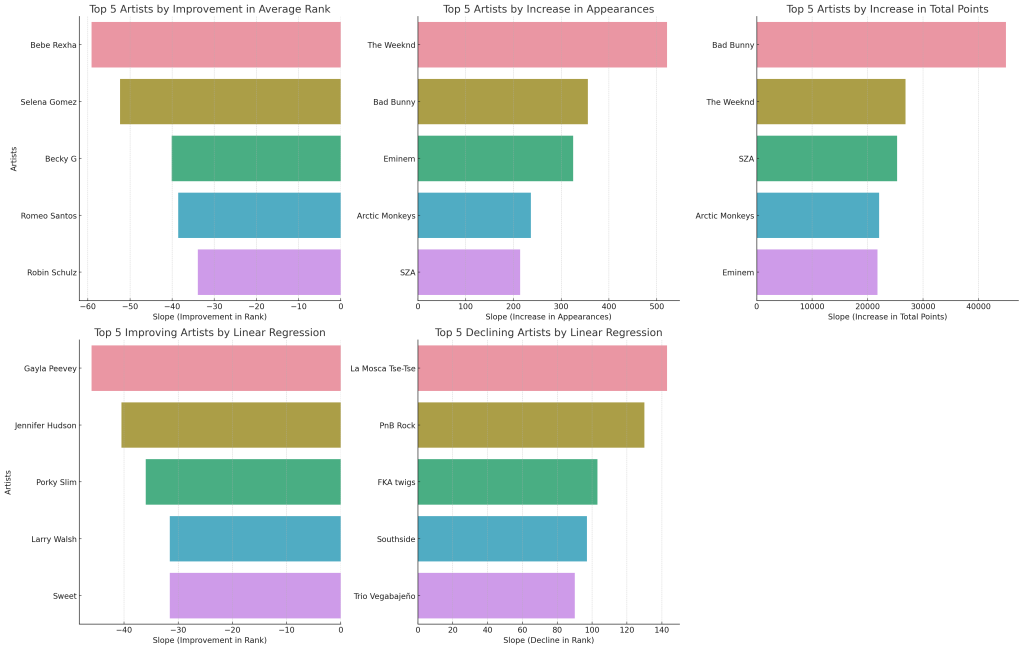
While the above data is based on individual contributions, we also checked on collaborations. The table below shows the top 5 collaborative artists based on unique titles in the dataset:
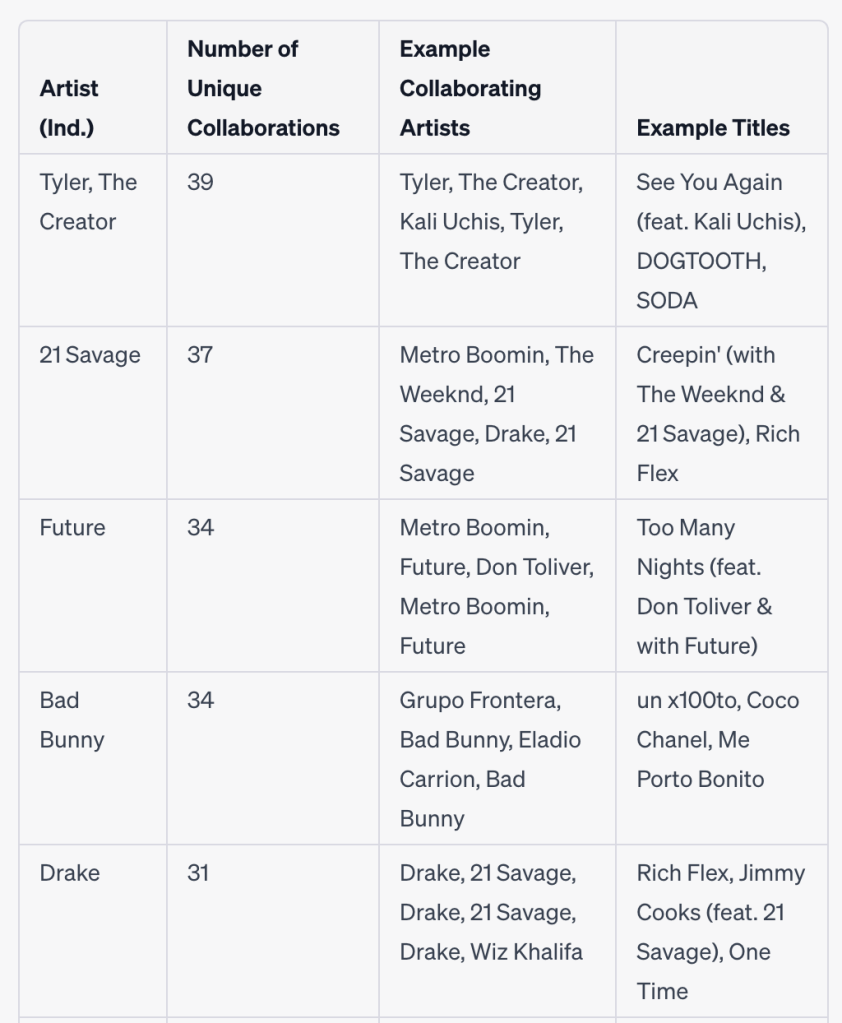
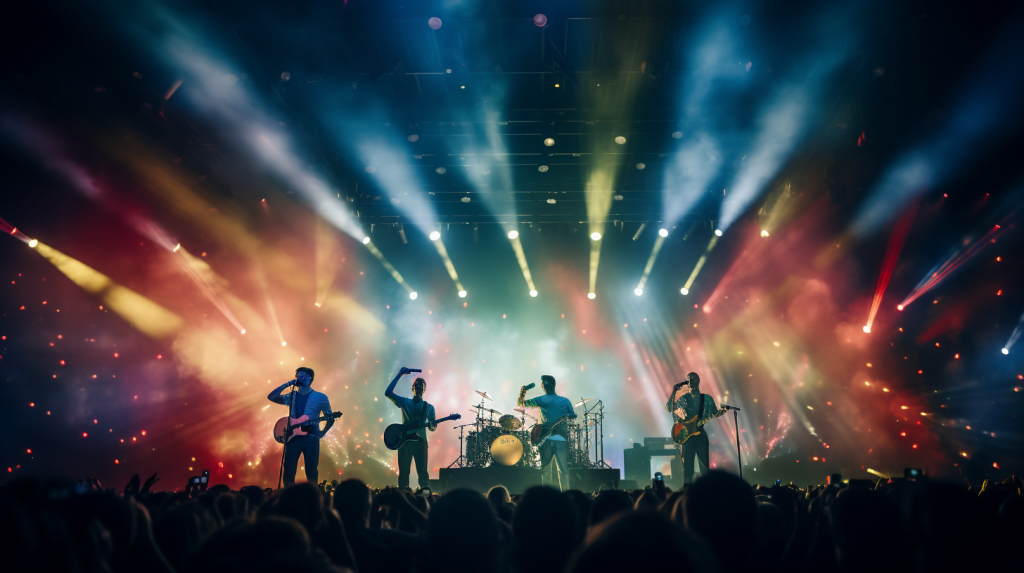
Consistency is Key: Artists and Long-Running Songs
When it comes to staying power, some artists have consistently appeared on the Top 200 lists over the past three years. Likewise, some songs have remarkably long lifespans on these charts. Here is a table presenting the top 10 longest-running titles on the Top 200 list, along with the corresponding artist(s) and the number of days they’ve been on the list:
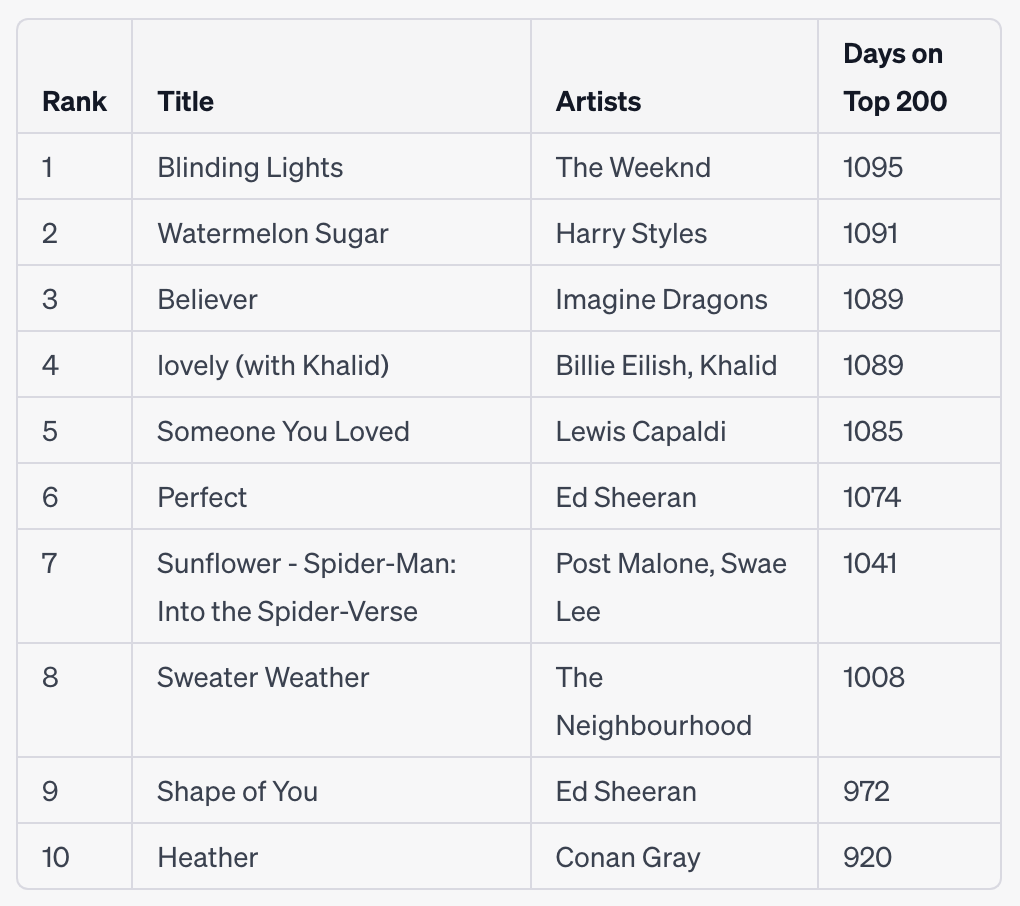
On the flip side, there are also 670 songs that have appeared only once on the Top 200 list during the three year period. Some examples:
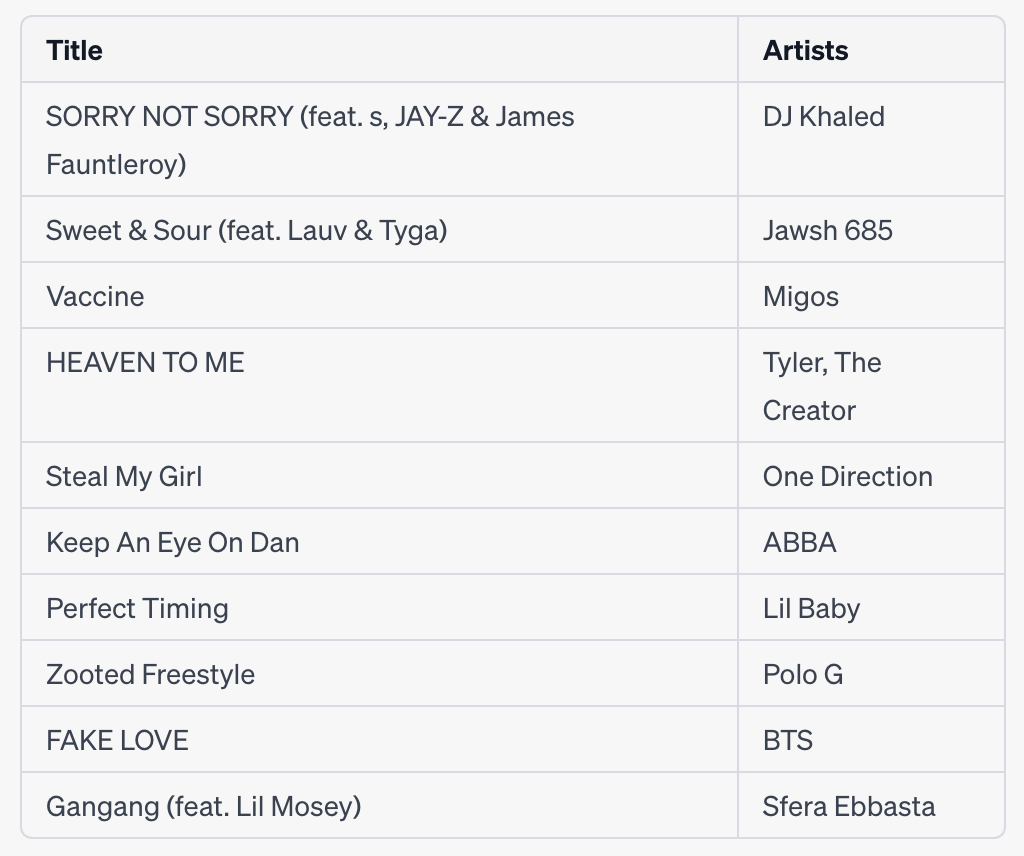
Geographical Dominance: Top Artists by Country and Continent
The dataset also reveals how artists from specific countries rule the charts. For example, here’s the top artist in each continent based on nationality:
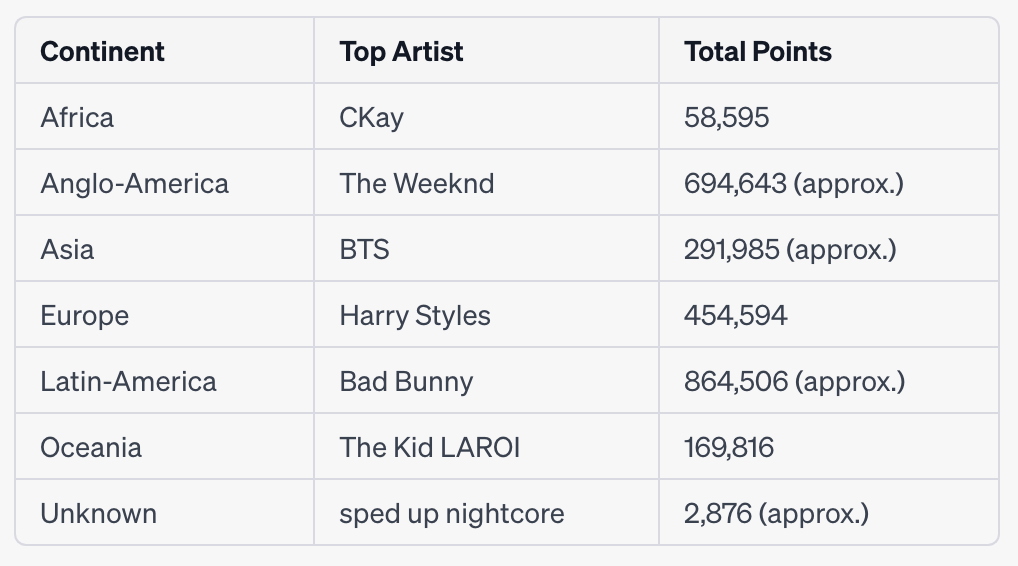
Based on a Country level analysis, the United States clearly dominates, followed by the United Kingdom and Canada. This perhaps isn’t surprising given the global influence of artists from these countries. However, it’s interesting to see other nations like South Korea and Colombia also making a mark on the global stage.
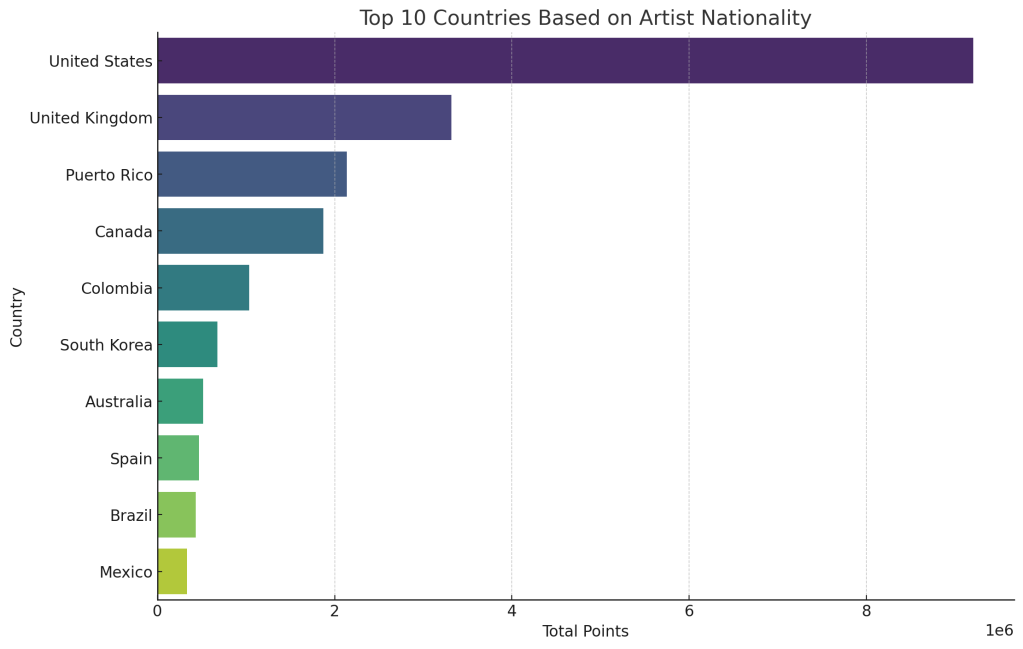
…and here are the top 5 artists from the United States, United Kingdom, Puerto Rico, and Canada based on their total individual points in the dataset.
United States
- Olivia Rodrigo: 480,992 Points
- Taylor Swift: 444,740 Points
- Doja Cat: 385,708 Points
- Billie Eilish: 342,073 Points
- Juice WRLD: 271,186 Points
United Kingdom
- Harry Styles: 454,594 Points
- Dua Lipa: 390,573 Points
- Ed Sheeran: 386,230 Points
- Lewis Capaldi: 218,306 Points
- Adele: 161,354 Points
Puerto Rico
- Bad Bunny: 864,506 Points
- Rauw Alejandro: 256,814 Points
- Farruko: 109,986 Points
- Myke Towers: 99,698 Points
- Chencho Corleone: 75,282 Points
Canada
- The Weeknd: 694,643 Points
- Justin Bieber: 369,183 Points
- Drake: 307,869 Points
- Shawn Mendes: 98,002 Points
- Tate McRae: 94,297 Points
The Anatomy of a Hit Song: Audio Features
The series of line graphs below depict how various audio features—Danceability, Energy, Speechiness, Acousticness, Instrumentalness, and Valence—have evolved over the span of three years, from 2020 to 2023.
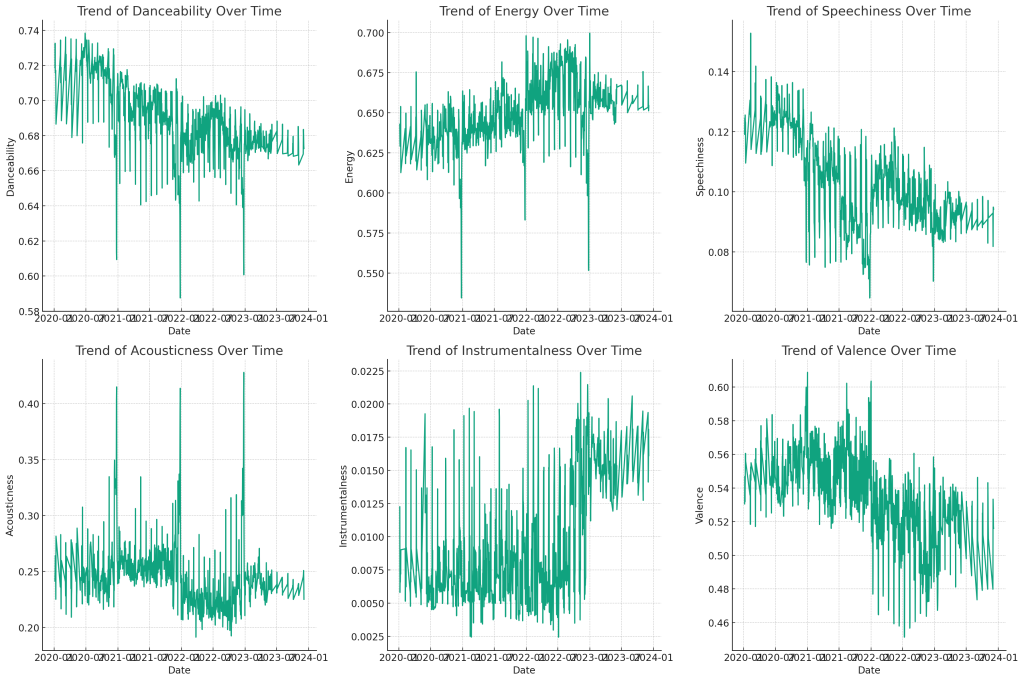
Key Takeaways:
- Danceability: There appears to be a slight increase in the danceability of songs, suggesting a trend towards more danceable music.
- Energy: The energy level of songs seems to have been relatively stable throughout the years, with only minor fluctuations.
- Speechiness: The presence of spoken words in tracks appears to have slightly increased, indicating a potential rise in genres like rap and spoken word.
- Acousticness: The level of acousticness shows a downward trend, possibly indicating a shift towards more electronic or synthesized music.
- Instrumentalness: This feature remains quite low and stable, suggesting that vocal tracks continue to dominate.
- Valence: The measure of musical positiveness seems to be relatively stable, with some minor ups and downs.
What About Loudness?
After converting the Loudness data to a more standard decibel (dB) format, we noticed that the average loudness of songs has remained relatively stable over the past three years. This could be indicative of a broader trend in the music industry known as the “loudness war,” where songs are mastered at higher volumes to stand out.
Conclusion
Analyzing these Top 200 playlists over a 3 year period offers quite a unique window into global music trends. While individual tastes in music are incredibly diverse, certain patterns and trends do emerge at scale. Also check out the bonus insights and caveats below…
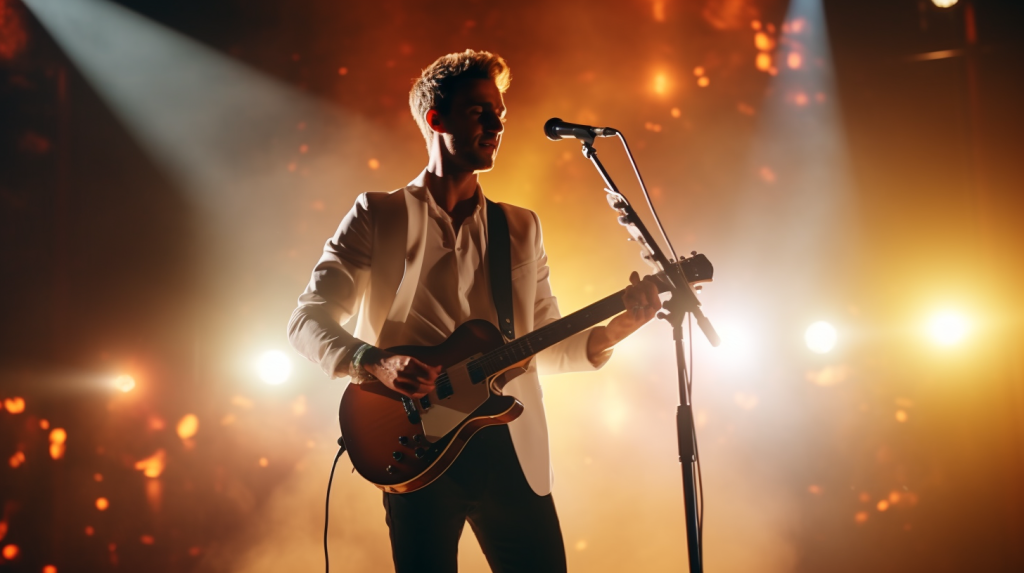
BONUS – A Personal Recommendation Engine
The great thing about having your hands on such a large dataset is the ability to find hidden gems and recommendations based on your personal preferences (similar to my Movie Recommendation Engine experiment).
Here are a couple of examples:
Prompt: Out of the previous top 20 artists list we created, let’s say I like songs by Taylor Swift, Coldplay and Imagine Dragons. Which of these artists’ songs have appeared on the top 200? Summarize by artist and sort in descending order in terms of how many days these songs have appeared on the list.
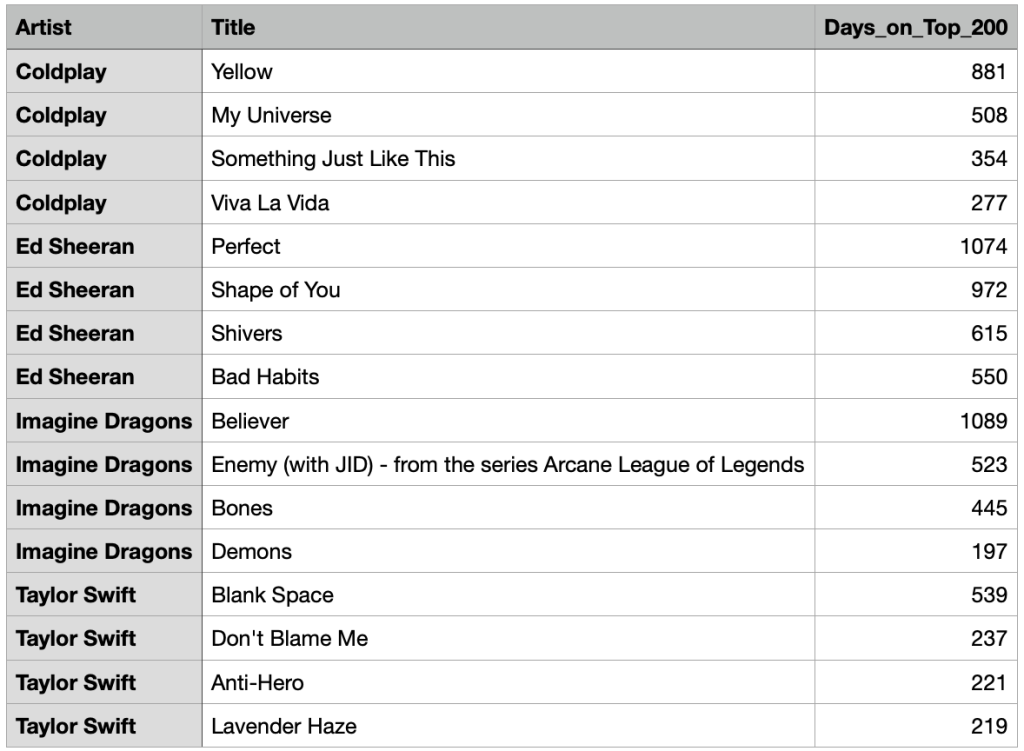
Prompt: I have a diverse taste in music, with a leaning towards rock and pop. Growing up in the 80’s and 90’s, I have a soft spot for popular artists and songs from these decades. While the release date for these titles isn’t provided in the dataset, can you use your own training to identify any older songs that still appear often in these rankings?
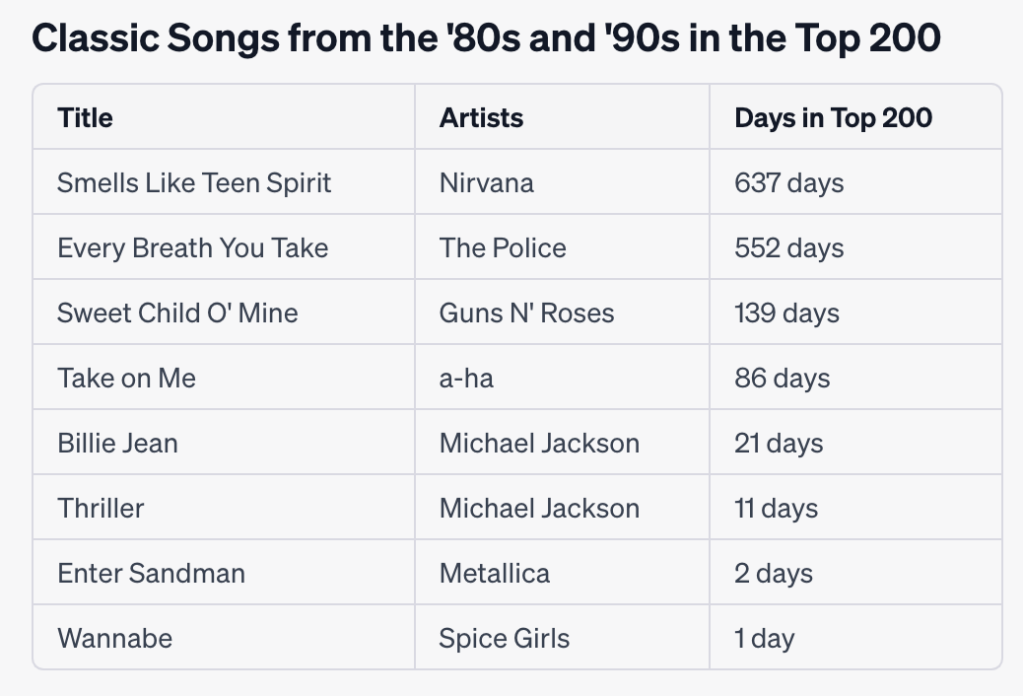
Prompt: Does Elton John have any collaborations that have featured in the charts?
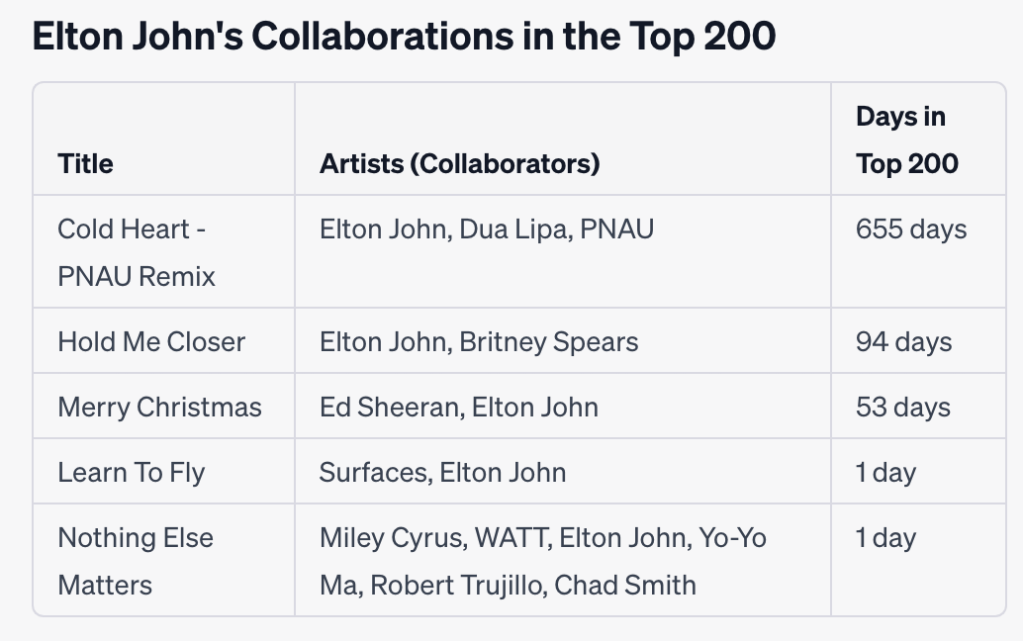
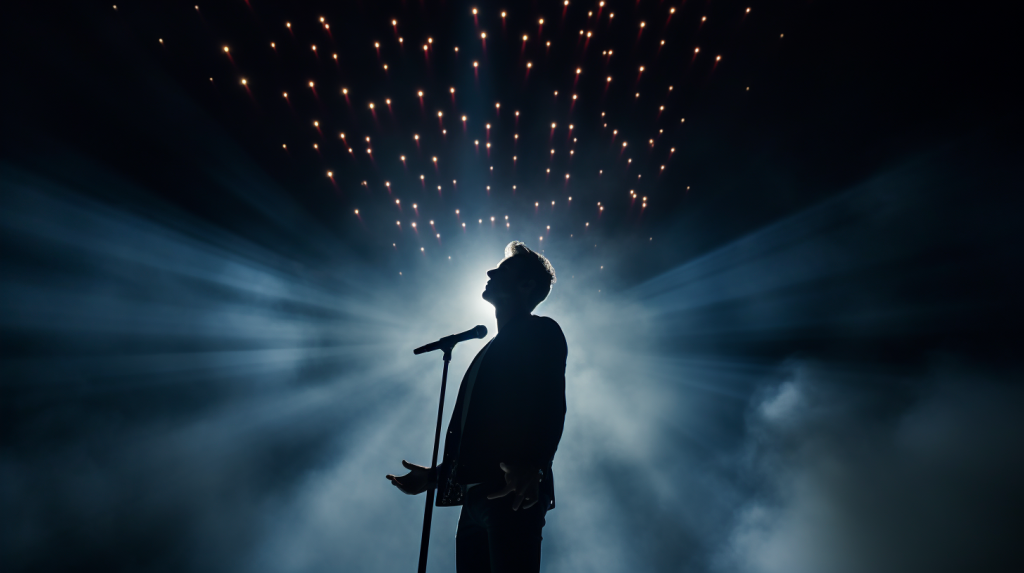
Potential Caveats and Drawbacks:
It’s important to note the following when looking at these results:
- Limited Scope: The dataset only includes songs from the “Top 200” playlist from ONE popular music streaming service, which may not fully represent broader musical tastes and trends.
- Short Time Span: The data covers a period of three years, which might not be long enough to identify long-term trends or shifts in the music industry.
- Age of Tracks: More recent releases may be at a disadvantage when looking at longevity on the charts.
- Geographic Bias: The dataset is globally aggregated, potentially overlooking regional or country-specific trends and preferences.
- Point Allocation: The point system, while useful for ranking, is arbitrary and might not accurately capture the essence of a song’s or artist’s popularity.
- Collaboration Complexity: For songs with multiple artists, the dataset evenly distributes points, which may not reflect the actual contribution of each artist to the song.
- Audio Features: While the audio features provide a good overview of a song’s musical properties, they are algorithmically generated and might not fully capture the subjective experience of listeners.
- Missing Data: The dataset might not include all relevant variables, such as genre, that could provide a more comprehensive analysis.
- Streaming vs. Sales: The data is based on streaming numbers and does not include other metrics like album sales, concert tickets, or merchandise, which also contribute to an artist’s popularity.
- Cultural Context: The dataset lacks context for why certain songs or artists may be popular, missing out on cultural, seasonal, or event-driven factors that could drive trends.
- Data Cleaning: Although the dataset is described as meticulously cleaned, there is always the potential for errors or omissions that could impact the analysis.
- ChatGPT + Code Interpreter Limitations: Through experimenting, it is evident that for specific analyses you have to be very particular in how you focus your prompts and queries…plus fact check the output from ChatGPT. The tool is powerful but by no means perfect.
What do you think? What other insights can the data provide? Anything you particularly loved or have questions about? Leave a comment or DM me to let me know.
0 comments on “ChatGPT Crunched 305,989 Song Rankings Over 1,095 Days to Uncover What’s Really Hot in Music”